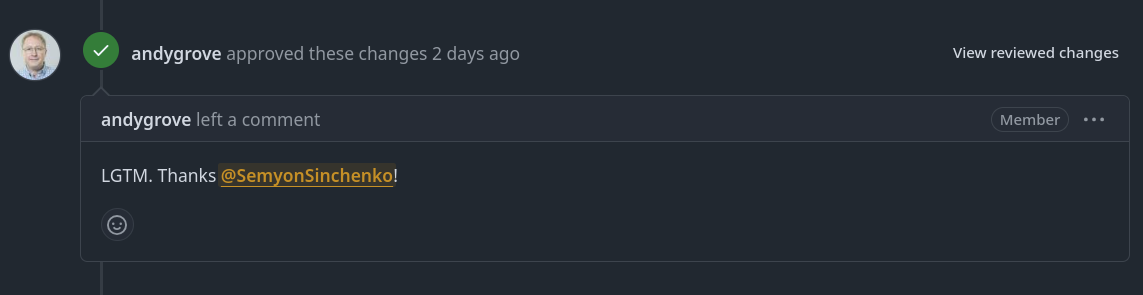
Apache Datafusion Comet and the story of my first contribution to it
In this blog post, I will provide a brief high-level overview of projects designed to accelerate Apache Spark by the native physical execution, including Databricks Photon, Apache Datafusion Comet, and Apache Gluten (incubating). I will explain the problems these projects aim to solve and their approaches. The main focus will be on the Comet project, particularly its internal architecture. Additionally, I will share my personal experience of making my first significant contribution to the project. This will include not only a description of the problem I solved and my solution but also insights into the overall contribution experience and the pull request review process.