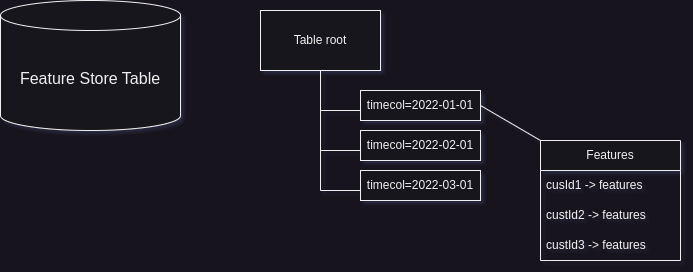
Effective asOfJoin in PySpark for Feature Store
Leveraging Time-Based Feature Stores for Efficient Data Science Workflows In our previous post, we briefly touched upon the concept of ML feature stores and their significance in streamlining machine learning workflows. Today, we’ll again explore a specific type of feature store known as a time-based feature store, which plays a crucial role in handling temporal data and enabling efficient feature retrieval for data science tasks. In this post we’ll how a feature-lookup problem may be effectively solved in PySpark using domain knowledge and understanding how Apache Spark works with partitions and columnar data formats....